The word statistics is derived from the Latin word “status” which means a state. Statistics normally means taking the values of some parameters and by plotting it or by arranging it in a meaningful manner. Basically, statistics give us the process by which we can collect, analyze, interpret, present and organize data.
In statistics, we normally use words like “mean”, ”median” and “mode” to understand the data distributed throughout a range. Be it grouped or ungrouped, usually, these central tendency measures give a single data point which typically shows the behaviour of the whole data.
Also, Check:
Previous Years IIT JEE Questions on Statistics
Types of Averages
By averages, we normally mean the mean, median and mode of any data set.
MEAN
Mean is the simple average of a data set, i.e., mean can be represented by:
In the mathematical sense, mean is called average, but the two words don’t mean the same, all the time.
Let us take an example:
(In the case of discrete data)
The marks obtained by 10 students in a class are 56, 54, 89, 74, 23, 16, 94, 52, 68, 100.
So, the mean of the data presented will be given by:
= 626/10
= 62.6
Now, let us take the case of grouped data.
The table shows the marks obtained by 10 students will be:
Marks | No. of students | Mid-point(x) | Value(x) |
0-10 | 1 | 5 | 5 |
11-20 | 5 | 15.5 | 77.5 |
21-30 | 2 | 25.5 | 51 |
31-40 | 3 | 35.5 | 106.5 |
41-50 | 6 | 45.5 | 273 |
51-60 | 8 | 55.5 | 444 |
61-70 | 1 | 65.5 | 65.5 |
71-80 | 5 | 75.5 | 377.5 |
81-90 | 4 | 85.5 | 342 |
91-100 | 2 | 95.5 | 191 |
1st Step: We have to find the mid-points of every class and the interval is 10.
2nd Step: It will be the multiplication of the midpoint by the class frequency.
3rd Step: Then we sum the frequency and divide it by the frequency to get the mean.
= 1933/10
= 193.3
MEDIAN
Median basically means the middle-most value in a data set. In case of an ungrouped data, firstly we will arrange the entire data set in ascending order. Now, if the number of terms is odd, we find the median by finding out the value of ((n+1)/2)th term and if the number of terms is even, the median will be given by the average of (n/2)th term and ((n+1)/2)th term
Median calculation for grouped data:
Step 1: Find the class mark xi for each class.
Step 2: Find N = ∑ fi
Step 3: Take the median class to be that value whose cumulative frequency is near about (N/2)
Step 4: Calculate the median by the following value:
l = lower limit of the median class
f = frequency of the median class
h = width of the median class
c = cumulative frequency of the class just preceding the median class
MODE
By mode, we usually represent the maximum occurrence of a single element in a series of elements. This also represents a measure of central tendency. Sometimes, a series has only elements with an occurrence of one time only, some have zero occurrences, and some have more than one.
Video Lesson: Mean, Median and Mode
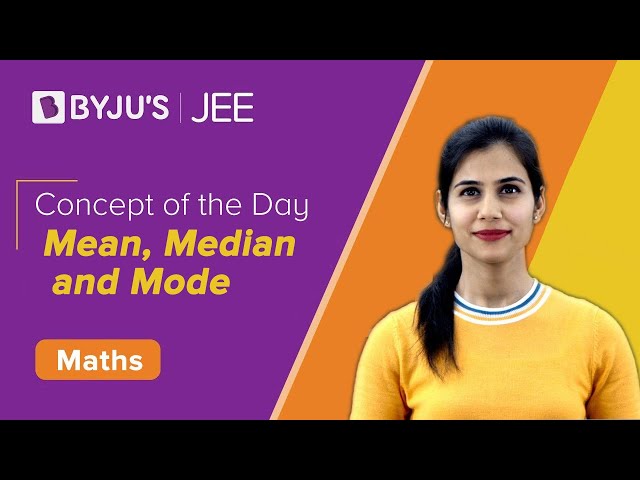
Dispersion in Statistics
By dispersion, we normally mean the extent to which the data is distributed or spread. It usually gives us a measure of the variation of every single data point from the average of all the points in a data set.
Now we can calculate dispersion by two types:
- Absolute value of the dispersion
- Relative value of the dispersion
Absolute Value of Dispersion
This takes into account the expression of the data with respect to the original data. They cannot be used to compare two or more data sets and tell us whether the data is highly scattered or not.
This includes:
|
Range:
We normally find the range by subtracting the minimum value from the maximum value in a data set. We cannot tell how much the data are dispersed or scattered- so, it is better that we introduce standard, mean and quartile deviation.
Mean Deviation:
The mean deviation tells us about the deviation from the mean or median data.
The formula of mean deviation is given by:
Variance
Sometimes we have to take the mean deviation by taking the absolute values from a set of values. The absolute values were taken to measure the deviations, as otherwise, the positive and negative deviation may cancel out each other.
So, to remove the sign of deviation, we usually take the variance of the data set, i.e., we usually square the deviation values. As squares are always positive, so the variance is always a positive number.
Let us take “n” observations as a1, a2, a3, ….., an
Then the variance is denoted by
Properties of Variance
- If the variance comes out to be zero, this means that \(\begin{array}{l}(a_i – \bar{a})\ \text{is equal to zero, }\end{array} \)\(\begin{array}{l}\text{which is nothing but each value of the set is equal to the mean value}\ \bar{a}.\end{array} \)
- If the variance is small, \(\begin{array}{l}\text{it means that the observations are pretty close to the mean value}\ \bar{a}.\end{array} \)If the value is greater,\(\begin{array}{l}\text{the deviations of the observations are far from the mean value}\ \bar{a}.\end{array} \)
- If each observation is increased by a where a ∈ R, then the variance will remain unchanged.
- If each observation is multiplied by a where a ∈ R, then the variance will be multiplied by a2 also.
- But for some data sets, the variance by the formula \(\begin{array}{l}\sum_{i=1}^n (a_i – \bar{a})^2\end{array} \)does not give the proper values as the range of deviation may vary, and the observations may be more scattered about the mean. So, to overcome this difficulty, we take the mean of the square of the deviations.
So, the variance is given by:
As a result of squaring, the unit of variance is not the same as that of the data sets taken.
Standard Deviation
To take a proper measure of dispersion, we have to calculate the standard deviation by taking the square root of the variance. This measure often prevents above-average deviations from cancelling those below, which can sometimes contribute to a null variance. If the variance is great, then the standard deviation will be more, and for lesser variance, the opposite case occurs.
The formula of standard deviation is given by:
Standard Deviation of distribution with discrete frequency:
It is given by:
Where the values are: a1, a2, a3 ,…., an
And the respective frequencies are: f1, f2, f3 ,…., fn
And
Standard Deviation of distribution with continuous frequency:
Relative Value of Dispersion
By this method, we represent the scattering in terms of some values, i.e., either in percentage or in the form of a ratio. This is mainly helpful to compare three or more data sets simultaneously.
The different forms of the relative measures of dispersion are:
● Coefficient of Range ● Coefficient of Mean Deviation ● Coefficient of Variance and Standard Deviation |
Coefficient of Range
It is defined as the ratio of the difference between the highest and lowest values to the sum of the two.
It is given by;
Coefficient of Mean Deviation
It is defined as the ratio of the mean deviation to the mean of the same data set.
It is given by (Mean deviation from Mean)/Mean or (Mean deviation from Median)/Median
Solved Examples
Example 1: An experiment is conducted with 16 values of b, and the following results were obtained. ∑ b2 = 2560 and ∑ b = 180. On checking through the data again, it is seen that one observation with a particular value 30 is replaced with 20. What will be the corrected variance?
Solution:
Given: ∑ b2 = 2560 and ∑ b = 180
So, ∑ b1 = 180 – 30 + 20 = 170
And the variance will be decreased by ∑ b2 = 900 – 400 = 500
The value of variance becomes ∑ b2 = 2560 – 900 + 400 = 2060
So, the corrected variance = 1/n ∑ (b2 -[1/n ∑ b1]2 )
= (1/16) × 2060 – (1/16 × 170)2
= 128.75 – 112.890625
= 15.859375
Example 2: Calculate the median for the following data:
Marks | 0-10 | 10-20 | 20-30 | 30-40 | 40-50 |
No. of Students | 5 | 15 | 20 | 2 | 8 |
Solution: First, we will calculate the cumulative frequency and mid-point.
Marks | Frequency | Cumulative frequency | Mid-point |
0-10 | 5 | 5 | 5 |
10-20 | 15 | 20 | 15 |
20-30 | 20 | 40 | 25 |
30-40 | 2 | 42 | 35 |
40-50 | 8 | 50 | 45 |
Thus, N = 50 and N/2 = 25
So, the median class is 20-30.
l = 20, f = 20, h = 10 and c = 20
So, median = 20 + [(25 – 20)/20] × 10 = 22.5
Example 3:
Let us take two sets of values where one set is represented by the scores of 100 Indian batsmen, and the other represents the scores of 100 Australian batsmen. Incidentally, the Indians have scored runs in the order 550, 551, 552, ….., 649. And the Australian batsmen have scored runs in the order 900, 901, 902, …., 999. If the variances of the two sets are represented by σA and σB, then what will be the value of σA /σB?
Solution:
We know,
Here, both the Australian and Indian batsmen have 100 consecutive positive integers and the value of n = 100, which is also the same.
So, σA /σB =1
Example 4: The S.D. of a variate x is s. The S.D. of the variate (ax + b)/c, where a, b, c are constant, is
Solution:
Let
where
Example 5: Let r be the range and
Solution:
We have
Now
Example 6: The mean and S.D. of the marks of 200 candidates were found to be 40 and 15, respectively. Later, it was discovered that a score of 40 was wrongly read as 50. The correct mean and S.D., respectively, are
A) 14.98, 39.95
B) 39.95, 14.98
C) 39.95, 224.5
D) None of these
Solution:
Example 7: The average of n numbers x1, x2, x3, …., xn is M. If xn is replaced by x’, then the new average is
Solution:
Hence, the new average
Statistics – Important Topics
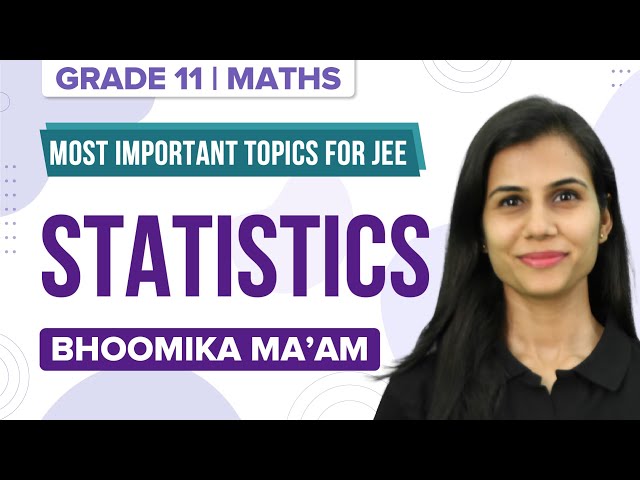
Statistics for IIT JEE in One-Shot
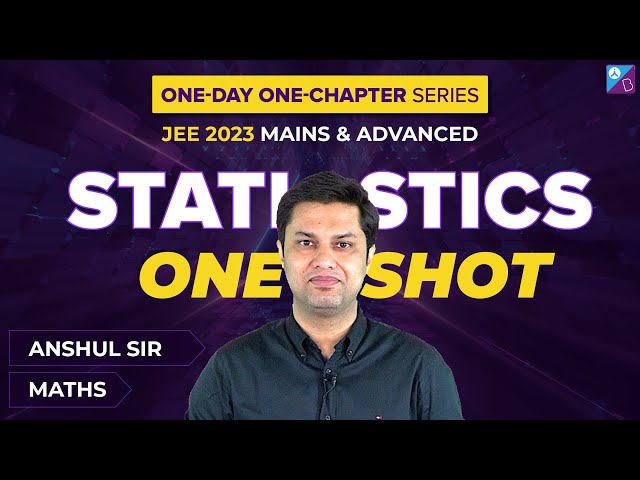
Comments