Mean and variance are measures of central dispersion. Mean is the average of a given set of numbers. The average of the squared difference from the mean is the variance.
Central dispersion tells us how the data that we are taking for observation are scattered and distributed. We will learn about different properties, but before that, we need to get familiar with some of the features like mean, median and variance of the given data distribution.
If we multiply the observed values of a random variable by a constant t, its simple mean, sample standard deviation and sample variance will be multiplied by t, |t| and t2, respectively. Also, if we add a constant m to the observed values of a random variable, that constant value will be added to the sample mean, but the sample standard deviation and sample variance remain unchanged. A similar rule applies to the theoretical mean and variance of random variables.
If Y = tx + m, then
\(\begin{array}{l}\mu_y = t \mu_x + m\end{array} \)
\(\begin{array}{l}\sigma^2_y = t^2 \sigma^2_x\end{array} \)
\(\begin{array}{l}\sigma_y = |t| \sigma_x\end{array} \)
Here, μx, σx2, and σx are the mean, variance and standard deviation of the random variable X, respectively, and μy, σy2, and σy are the mean, variance and standard deviation of the random variable Y, respectively. |
Mean in Statistics
The term average of a random variable in probability and statistics is the mean or the expected value. If we know the probability distribution for a random variable, we can also find its expected value. The mean of a random variable shows the location or the central tendency of the random variable.
The definition of ‘mean’ is different in different branches of Mathematics. Normally, by mean, we usually denote the average of the discrete data present in a set of numbers. The arithmetic mean is usually given by (This is the formula that we represent for ungrouped data),
\(\begin{array}{l}\bar{x} = \frac{x_1+x_2+x_3+……+x_n}{n}\end{array} \)
Or \(\begin{array}{l}\bar{x} = \frac{1}{n}\sum_{i=1}^n x_i\end{array} \)
Where x1, x2, x3, ….., xn denote the value of the respective terms, And n = number of terms |
Let us take another example where each data point is given with separate frequency data.
The formula for the mean calculation in this case (called the discrete frequency data) is,
\(\begin{array}{l}\bar{x} = \frac{f_1x_1+ f_2x_2+ f_3x_3+……+ f_nx_n}{n}= \frac{1}{n}\sum_{i=1}^n f_ix_i \end{array} \)
Where, x1, x2, x3, ….., xn denote the value of the respective terms And f1, f2, f3, ….., fn denote the respective frequency data of the respective term And n = number of terms |
The formula for both the sample and the population taken is the same, but the denotation is different; the sample mean is denoted by x̄, and the population mean is represented by μ.
Properties of Mean
Some properties of the mean are given by:
1. If we increase individual units by k, then the mean will increase by k.
2. If we decrease individual units by k, then the mean will decrease by k.
3. If we multiply each unit by k, then the mean will be multiplied by k.
4. If we divide each unit by k, then the mean will be divided by k.
What Is Variance in Statistics?
Variance is the expected value of the squared variation of a random variable from its mean value. Sometimes, we have to take the mean deviation by taking the absolute values from a set of values. The absolute values were taken to measure the deviations; otherwise, the positive and negative deviations may cancel out each other.
So, to remove the sign of deviation, we usually take the variance of the data set, i.e., we usually square the deviation values. As squares are always positive, the variance is always a positive number.
Let us take ”n” observations as a1, a2, a3,…..,an and their mean is represented by
Then, the variance is denoted by
Check: How to Find Variance
Variance of Random Variables in Probability and Statistics
The variance of a random variable shows the variability of the random variables. Variance represents the distance of a random variable from its mean.
It can be calculated by using below formula:
σx2 = Var (X) = ∑i (xi − μ)2 p(xi) = E(X − μ)2
Var(X) = E(X2) − [E(X)]2
[E(X)]2 = [∑i xi p(xi)]2 = μ and E(X2) = ∑i xi2 p(xi).Properties of Variance
(1) If the variance is zero, this means that
(2) If the variance is small, it means that the observations are pretty close to the mean value, and if the value is greater, the deviations of the observations are far from the mean value.
(3) If each observation is increased by ‘a’ where aϵR, then the variance will remain unchanged.
(4) If each observation is multiplied by ‘a’ where a ϵ R, then the variance will be multiplied by a2 also.
But for some data sets, the variance by the formula
So, the variance is given by:
Also, Read
Solved Examples
Question 1: An experiment is conducted with 16 values of b, and the following results were obtained: ∑ b2 = 2560 and ∑ b = 180. On checking through the data again, it is observed that one observation with a particular value 30 is replaced with 20. Then, the corrected variance will be
Solution:
∑ b2 =2560 and ∑ b=180
So, ∑ b1 = 180 – 30 + 20 = 170
And the variance will be decreased by
∑ b2 = 900 – 400 = 500
The value of variance becomes ∑ (b1)2=2560-900+400 = 2060
So, the corrected variance will be = 1/n ∑ (b1)2 – [1/n ∑ b1]2 = 1/16 × 2060 – (1/16 × 170)2 = 128.75 – 112.890625 = 15.859375
Question 2: Let us take two sets of values where one set is represented by the scores of 100 Indian batsmen, and the other represents the scores of 100 Australian batsmen. Incidentally, the Indians have scored runs in the order 550, 551, 552……649. And the Australian batsmen have scored runs in the order 900, 901, 902….999. If the variances of the two sets are represented by
σA and σB, then σA/σB is
Solution:
We know, σ2 = (∑ di2)/n
Here, both the Australian and Indian Batsmen sets have 100 consecutive positive integers and the value of n = 100, which is also the same. Thus, ∑ di2 is the same for both of these integer sets.
So, σA/σB =1
Question 3: Find the mean and variance of the new random variables if we are given the mean and variance of the random variable X are 125 and 225, respectively.
Solution: The new random variable is the original random variable minus its mean.
Let Y be another random variable, and then
Y = X – 125
If we create a new variable Z = Y/15, which is obtained by dividing the random Y by its standard deviation, the mean, variance and standard deviation of this new variable are
The new random variable Z has a mean of 0 and a variance of 1.
Question 4: The mean of the values 0, 1, 2,……,n having corresponding weight
Solution:
The required mean is
Question 5: The average of n numbers x1, x2, x3, …., xn is M. If xn is replaced by x’, then the new average is
Solution:
M = (x1 + x2 + x3 +…. + xn )/n
nM = x1 + x2 + x3 +…. + xn-1 + xn
nM – xn = x1 + x2 + x3 +…. + xn-1
Statistics – Important Topics
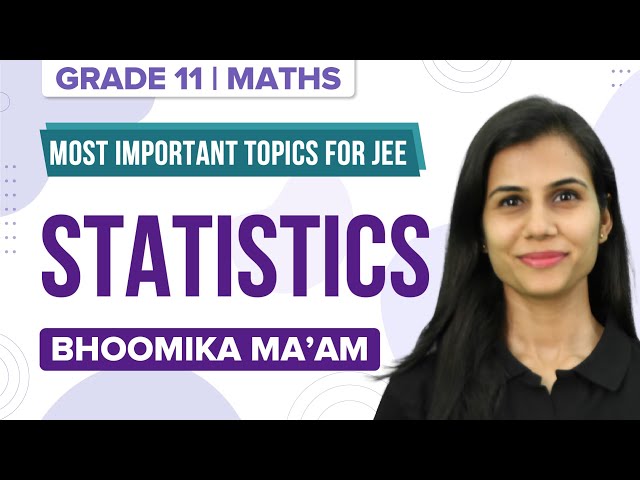
Mean, Median and Mode
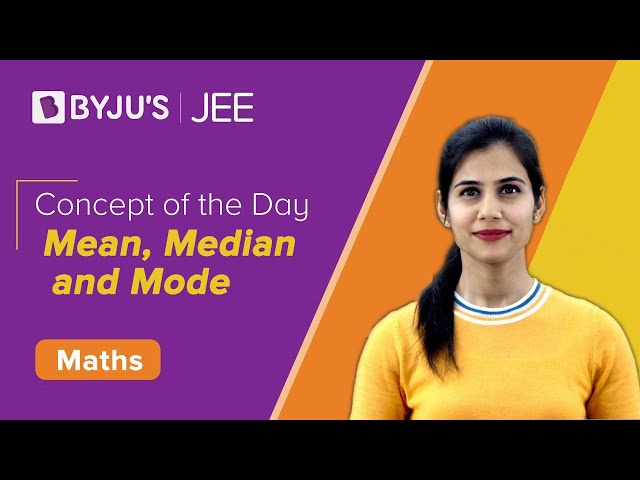
Frequently Asked Questions
What do you mean by variance?
The average of the squared differences from the mean is called variance. Variance is denoted by σ2.
What do you mean by mean in statistics?
Mean is the average of a given set of observations.
Give two properties of the mean.
If the individual units are increased by k, then the mean will increase by k.
If the individual units are decreased by k, then the mean will decrease by k.
Give the equation for finding the variance.
Variance is given by the equation σ2 = ∑(xi-x̄)2/N
x1, x2, …., xN are the N observations.
Comments